Blog
Prefect Product
Introducing Assets: From @task to @materialize
June 11, 2025


Workflow Orchestration
How to Cut Data Pipeline Costs by 75% with Kubernetes Spot Instances
June 2025

Case Studies
Kraft Analytics Group Platform Evolution with Prefect
May 2025

Prefect Product
How to Roll Back Deployments in Prefect with Deployment Versioning
May 2025

Prefect Product
Building Better Data Platforms with CI/CD
April 2025

Introducing Two New Self-Serve Plans for Prefect Cloud
April 2025

Introducing Prefect Serverless
April 2025

Case Studies
How WHOOP Cut Incidents by 75% with Prefect
April 2025

Case Studies
How an Enterprise Engineering Team Reduced Deployment Times from Hours to 20 Minutes with Prefect
April 2025

Prefect Product
Managing Prefect Deployments with Terraform
April 2025

Prefect Product
Beyond Loops: How Prefect's Task Mapping Scales to Thousands of Parallel Tasks
March 2025

Open Source Love Letters
How AnyIO Powers Prefect's Async Architecture
March 2025

Engineering
Switching a Big Python Library from setup.py to pyproject.toml
March 2025

Engineering
What Data Professionals Need to Know about uv
February 2025

Workflow Orchestration
Airflow to Prefect: Your Migration Playbook
February 2025

Workflow Orchestration
Airflow to Prefect: Why Modern Teams Choose Prefect
February 2025

Prefect Product
Why We Went All In on Type Completeness
January 2025

Workflow Orchestration
Building Real-Time Data Pipelines: A Guide to Event-Driven Workflows in Prefect
January 2025

Case Studies
How LiveEO Harnesses Petabytes of Satellite Data with Prefect
January 2025

Prefect Product
Prefect's Observability Suite
January 2025

Engineering
Stop Making Your Data Team Learn Kubernetes
January 2025

Workflow Orchestration
Don’t Buy a Fancy Cron Tool
January 2025

Events
Supercharging dbt: 3 Ways Prefect Enhances Your Data Workflows
December 2024

Press
Experimenting with Remote First: Humans Shouldn't Be Your Only Strategic Hires
December 2024

Workflow Orchestration
Push and Pull Architecture: Event Triggers Vs. Sensors in Data Pipelines
December 2024

Events
Four Infrastructure Trends Reshaping Modern Systems
November 2024

Case Studies
Modern Orchestration: Endpoint’s evolution from Airflow to Prefect
November 2024

Case Studies
Orchestrating Fashion's Data Runway: Inside Rent the Runway's Data Stack with Prefect and dbt
November 2024

Workflow Orchestration
The Importance of Idempotent Data Pipelines for Resilience
November 2024

Events
Building a Modular Data Architecture
November 2024

Prefect Product
From Chaos to Clarity: Managing Data Lakes with Prefect
November 2024

Workflow Orchestration
Built to Fail: Design Patterns for Resilient Data Pipelines
November 2024

Workflow Orchestration
Microservices Orchestration: What It Is, How to Use It
October 2024

Workflow Orchestration
The Hidden Factory of Long Tail Data Work
October 2024

Events
dbt Coalesce 2024 Takeways
October 2024

Workflow Orchestration
Every Company is a Data Processing Company
October 2024

Prefect Product
Break It, Fix It, Reverse It: Transactional ML Pipelines with Prefect 3.0
September 2024

Workflow Orchestration
Prefect on the lakehouse: write-audit-publish pattern with Bauplan
September 2024

OSS
Prefect's Commitment to Open Source: Launching Our Sponsorship Initiative
September 2024

AI
ControlFlow 0.9: Take Control of your Agents
September 2024

Workflow Orchestration
What I Talk About When I Talk About Orchestration
September 2024

Case Studies
How One Education Travel Company Unblocks Data Science Teams With Prefect
September 2024

Prefect Product
Prefect 3.0: Now Generally Available
August 2024

Workflow Orchestration
Orchestration Tools: Choose the Right Tool for the Job
August 2024

Case Studies
Cox Automotive Meets Dynamic Demands with Workforce Analytics Solutions Powered by Prefect
July 2024

Workflow Orchestration
When That Adhoc Script Turns Into a Production Data Pipeline
July 2024

Prefect Product
Background Tasks: Why They Matter in Prefect
July 2024

Case Studies
Cash App Gains Flexibility in Machine Learning Workflows with Prefect
July 2024

Engineering
Managing Efficient Technical and Data Teams
July 2024

Prefect Product
Introducing Prefect 3.0
June 2024

AI
Introducing ControlFlow
June 2024

Workflow Orchestration
Work Pools and Workers: Deploy Python Securely
June 2024

Prefect Product
5 Reasons You Can't Miss Prefect Summit 2024
June 2024
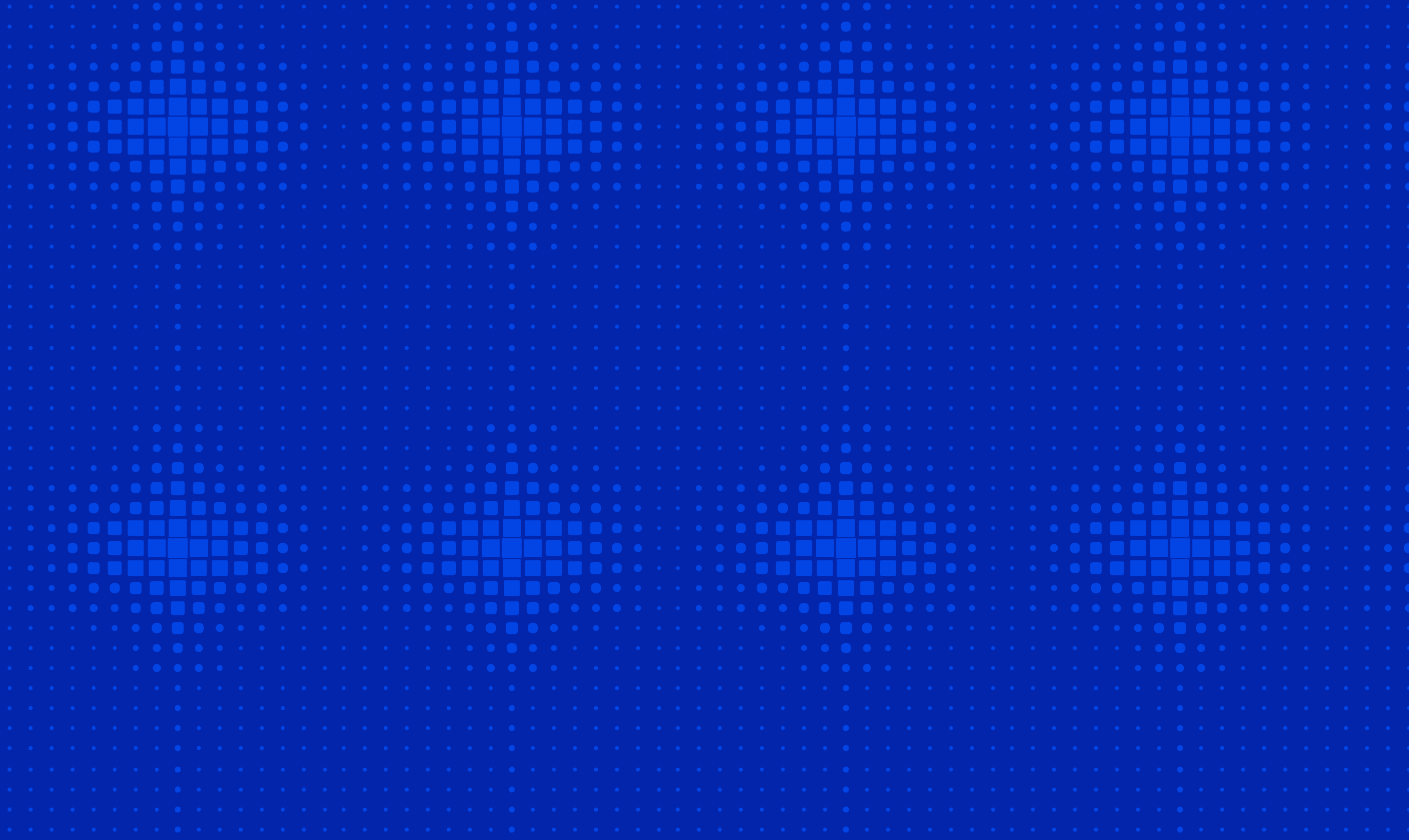
Notes
The Hidden Costs of Running Apache Airflow
June 2024

Engineering
The Role of Infrastructure Cleanup Jobs
May 2024
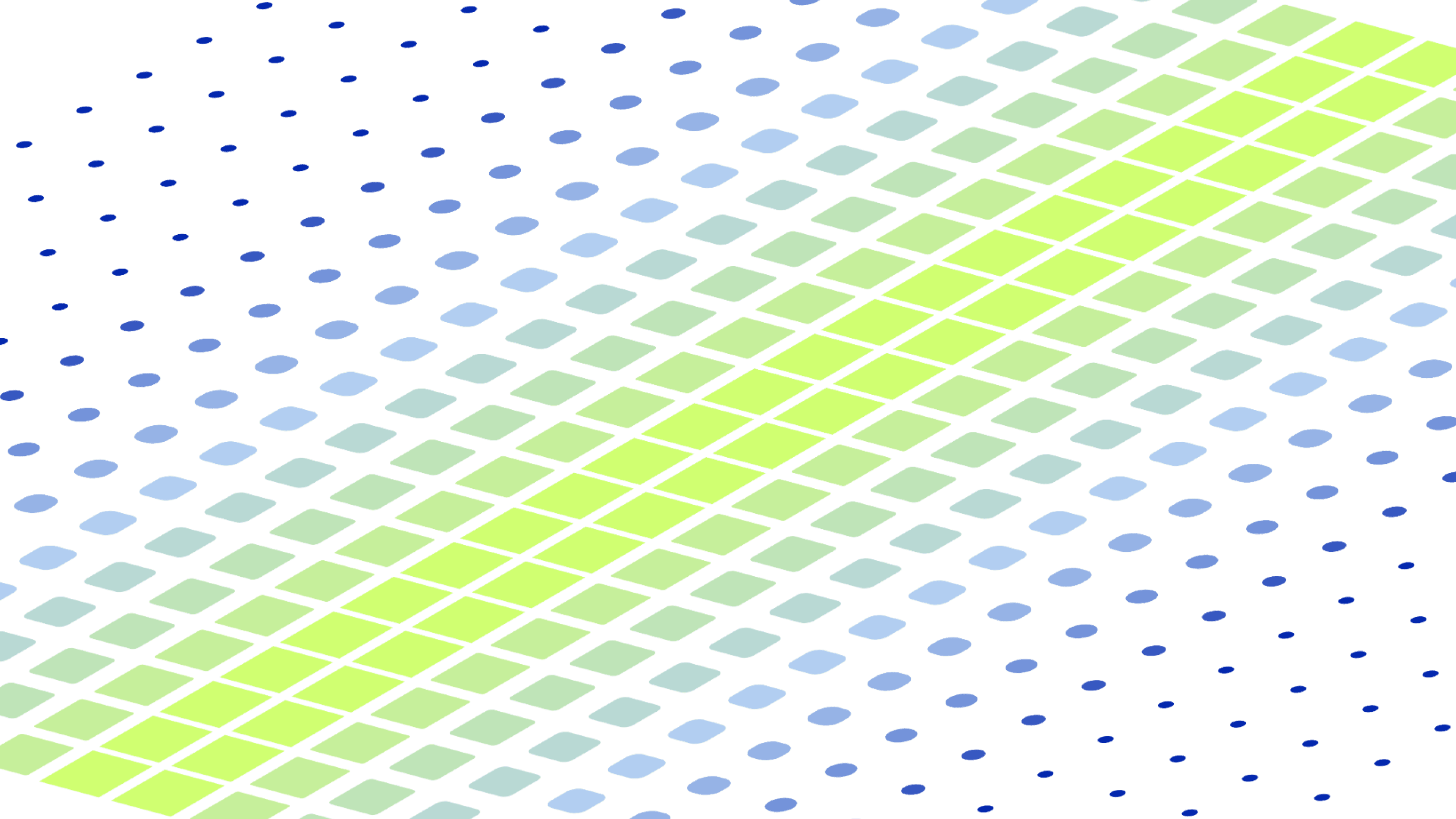
Engineering
Dockerizing Your Python Applications: How To
April 2024

Engineering
Data Validation with Pydantic
April 2024
Workflow Orchestration
Glue Code: How to Implement & Manage at Scale
April 2024
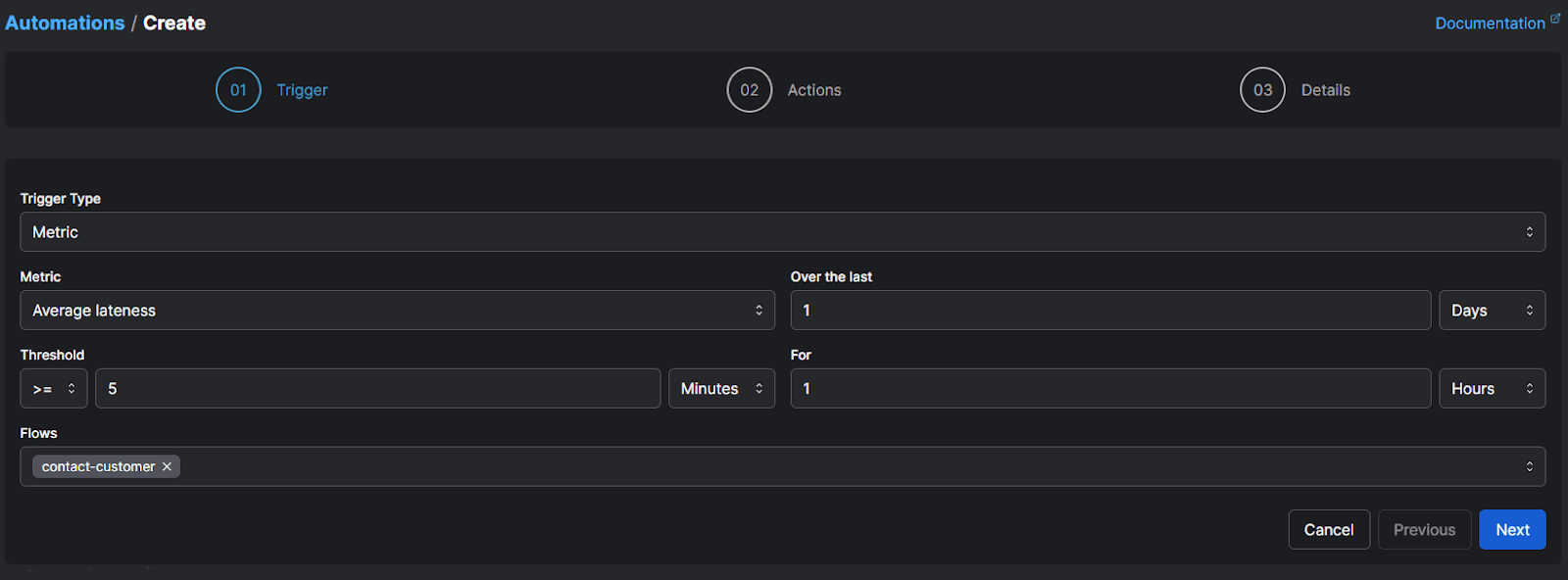
Workflow Orchestration
Observability Metrics: Put Your Log Data to Use
March 2024
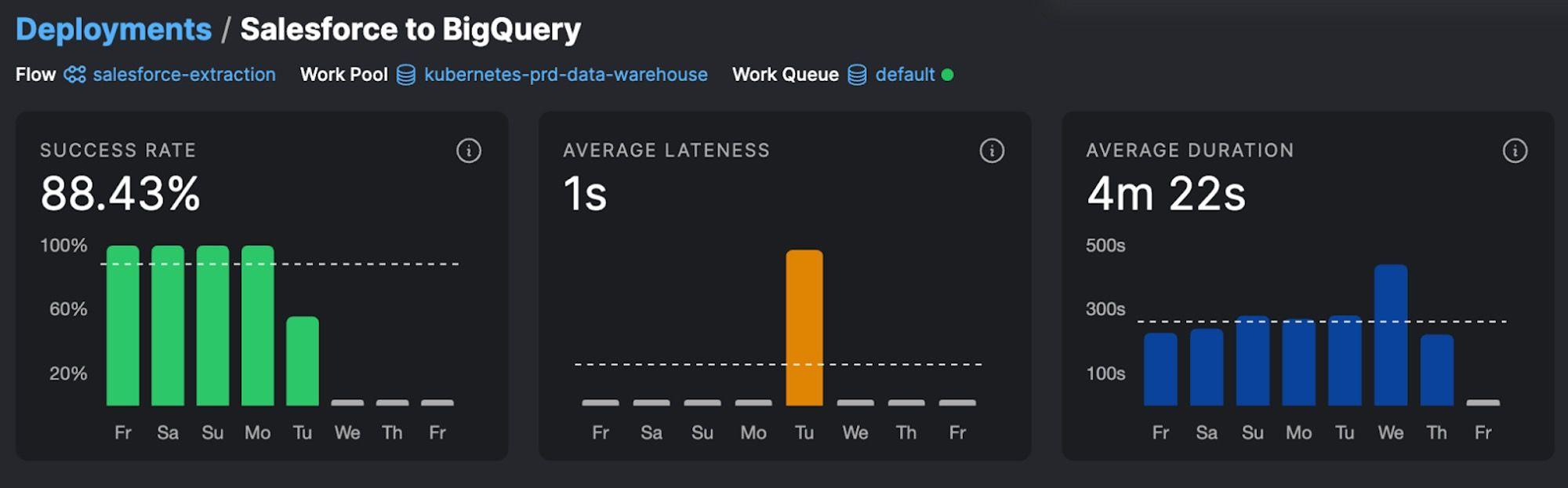
Workflow Orchestration
Data Pipeline Monitoring: Best Practices for Full Observability
March 2024
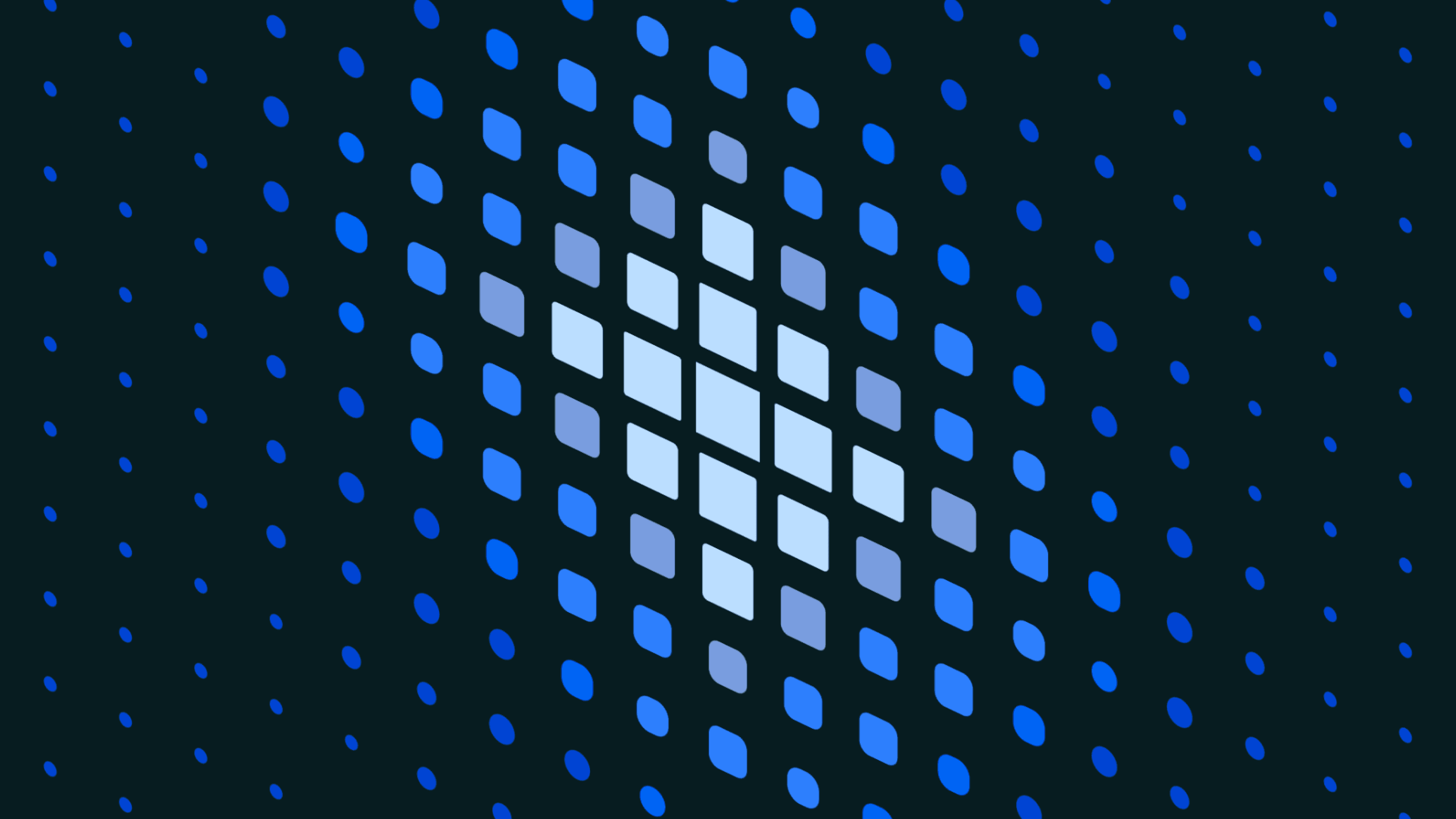
Engineering
Pydantic Enums: An Introduction
March 2024

Prefect Product
Orchestrating dbt on Snowflake with Prefect
February 2024
Workflow Orchestration
Workflow Observability: Finding and Resolving Failures Fast
February 2024
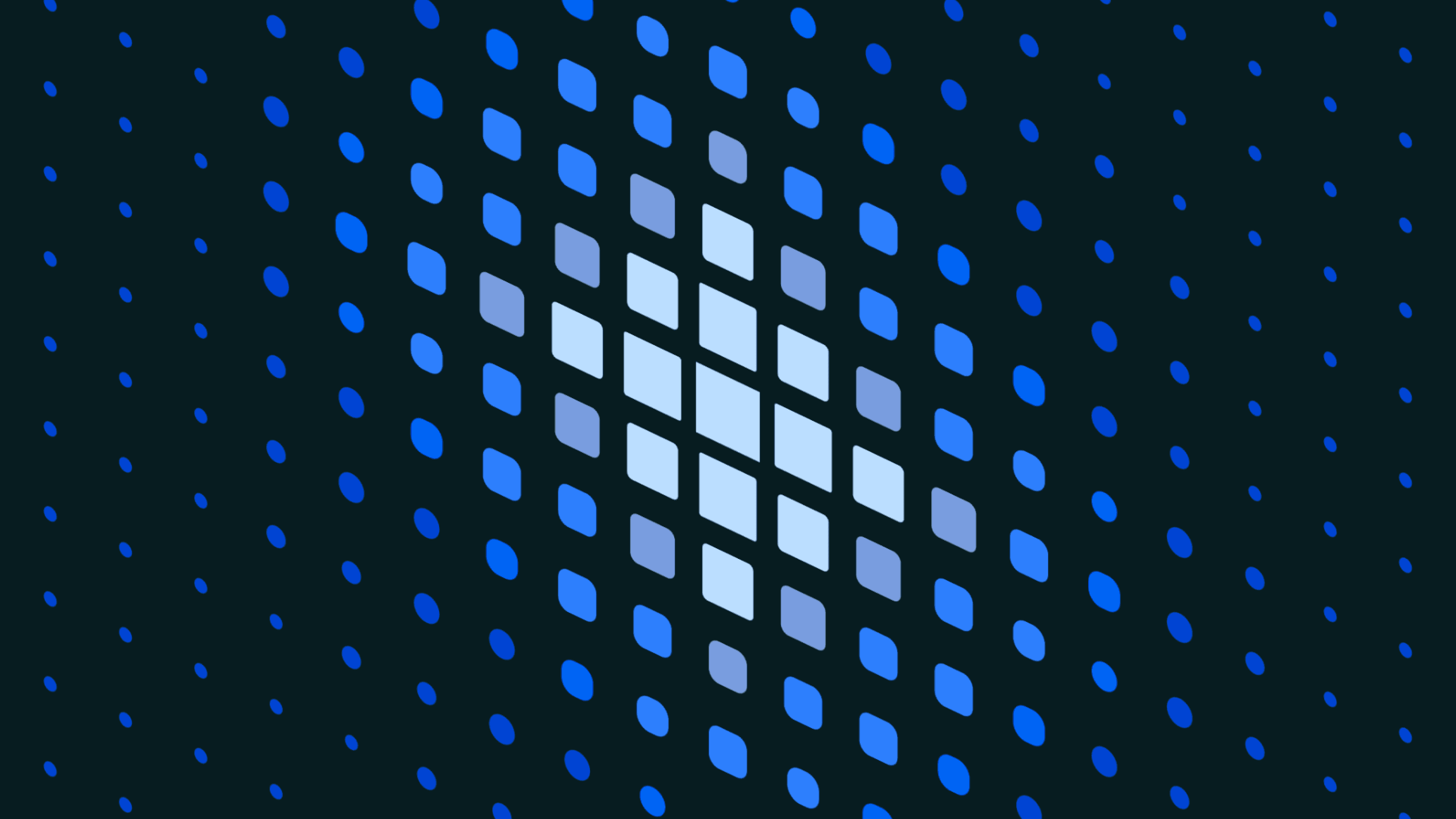
Engineering
What is Pydantic? Validating Data in Python
February 2024
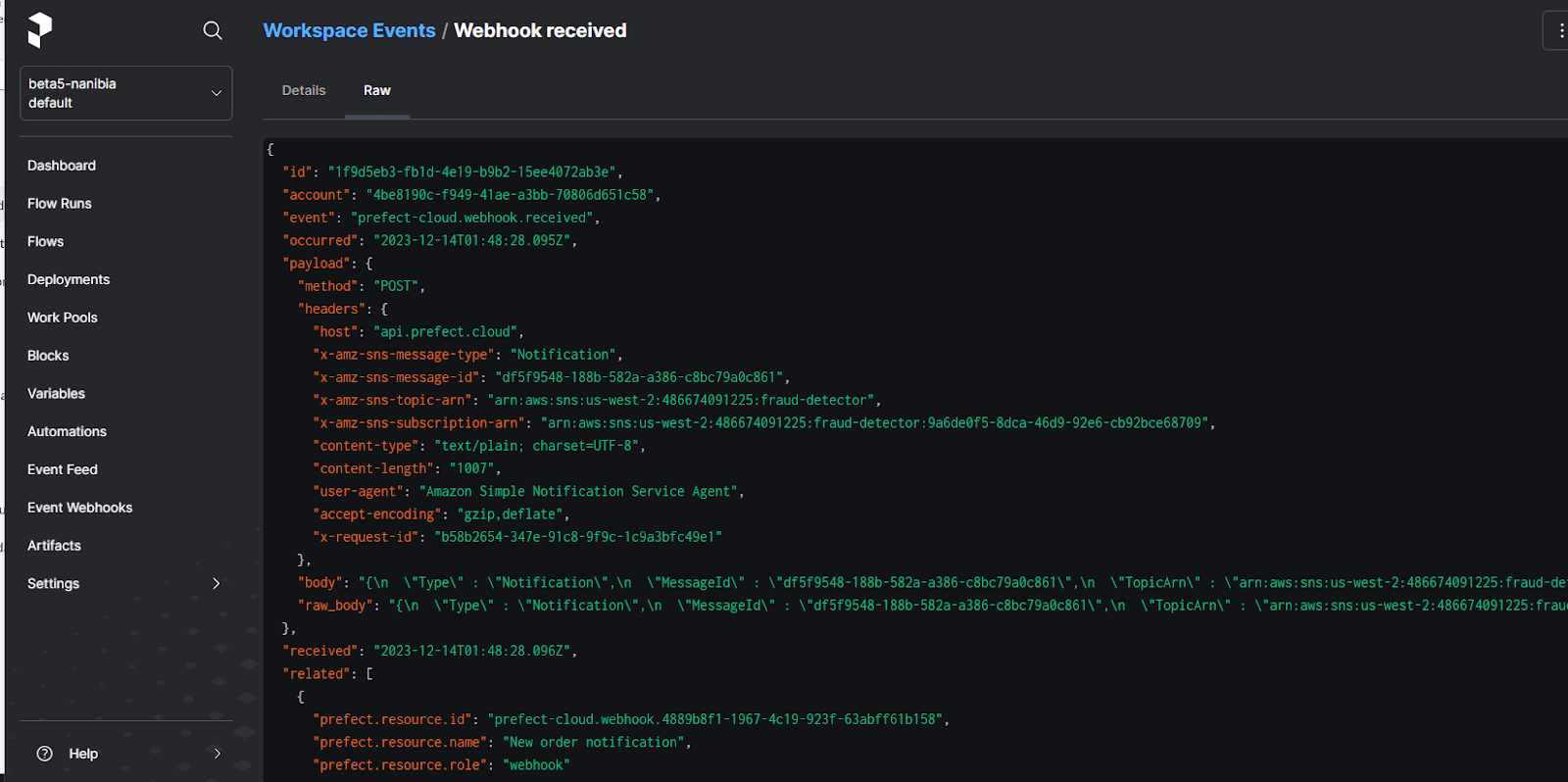
Workflow Orchestration
Event-Driven Versus Scheduled Data Pipelines
February 2024
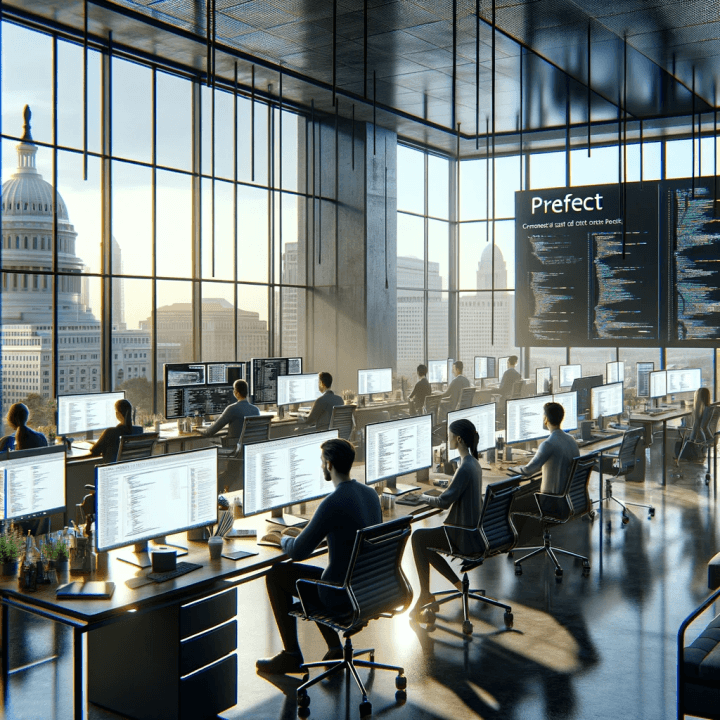
Press
How Jeremiah Lowin Turned a Life-Long Question Into an Industry-Leading Startup Based in D.C.
February 2024
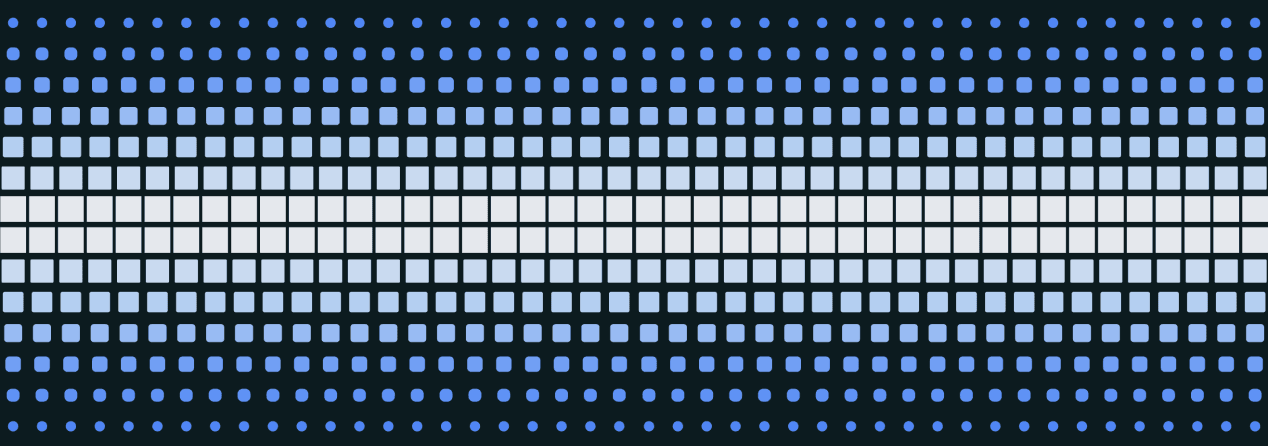
Prefect Product
Unveiling Interactive Workflows
January 2024
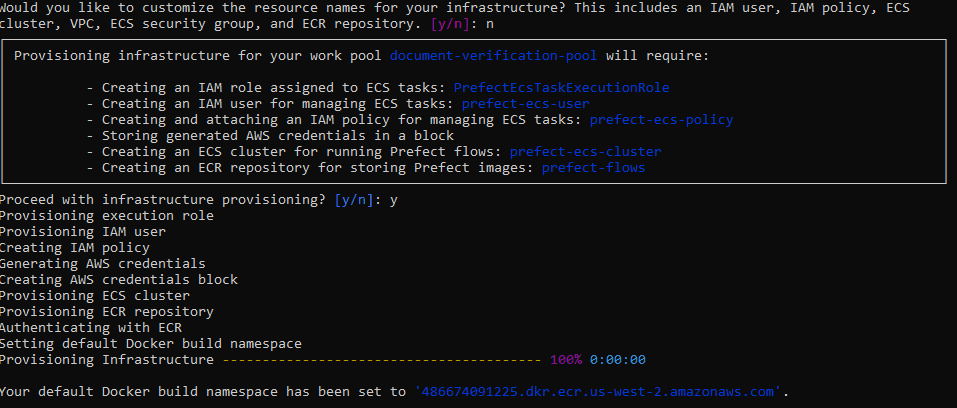
Workflow Orchestration
Scalable Microservices Orchestration with Prefect and Docker
January 2024
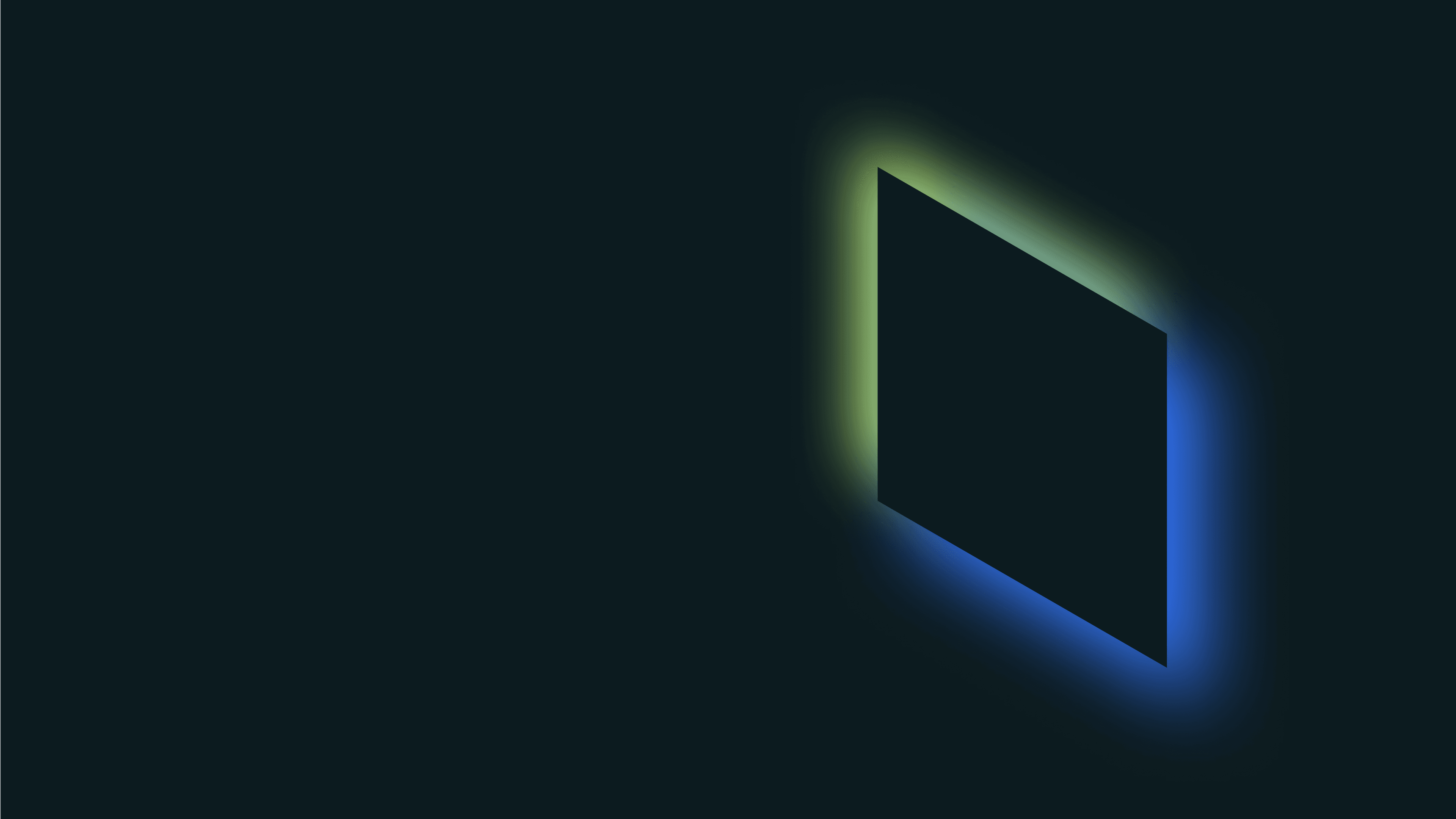
Prefect Product
Product updates: incident management, managed compute, and much more
January 2024

Prefect Product
Schedule Python Scripts
January 2024
Prefect Product
Reducing the Workflow Hangover in 2023
January 2024
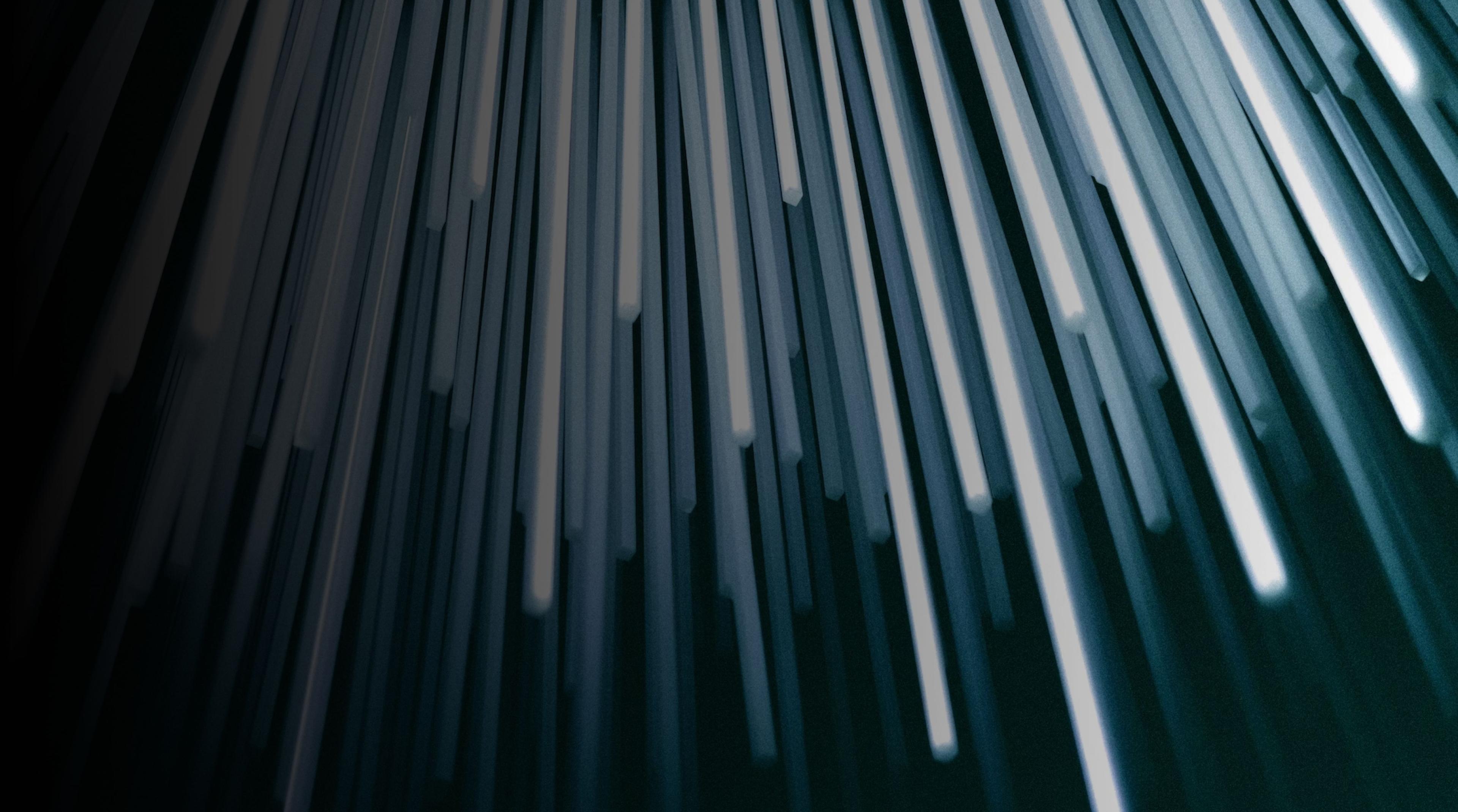
Workflow Orchestration
Orchestrating Event Driven Serverless Data Pipelines
January 2024
Workflow Orchestration
Why You Need an Observability Platform
December 2023
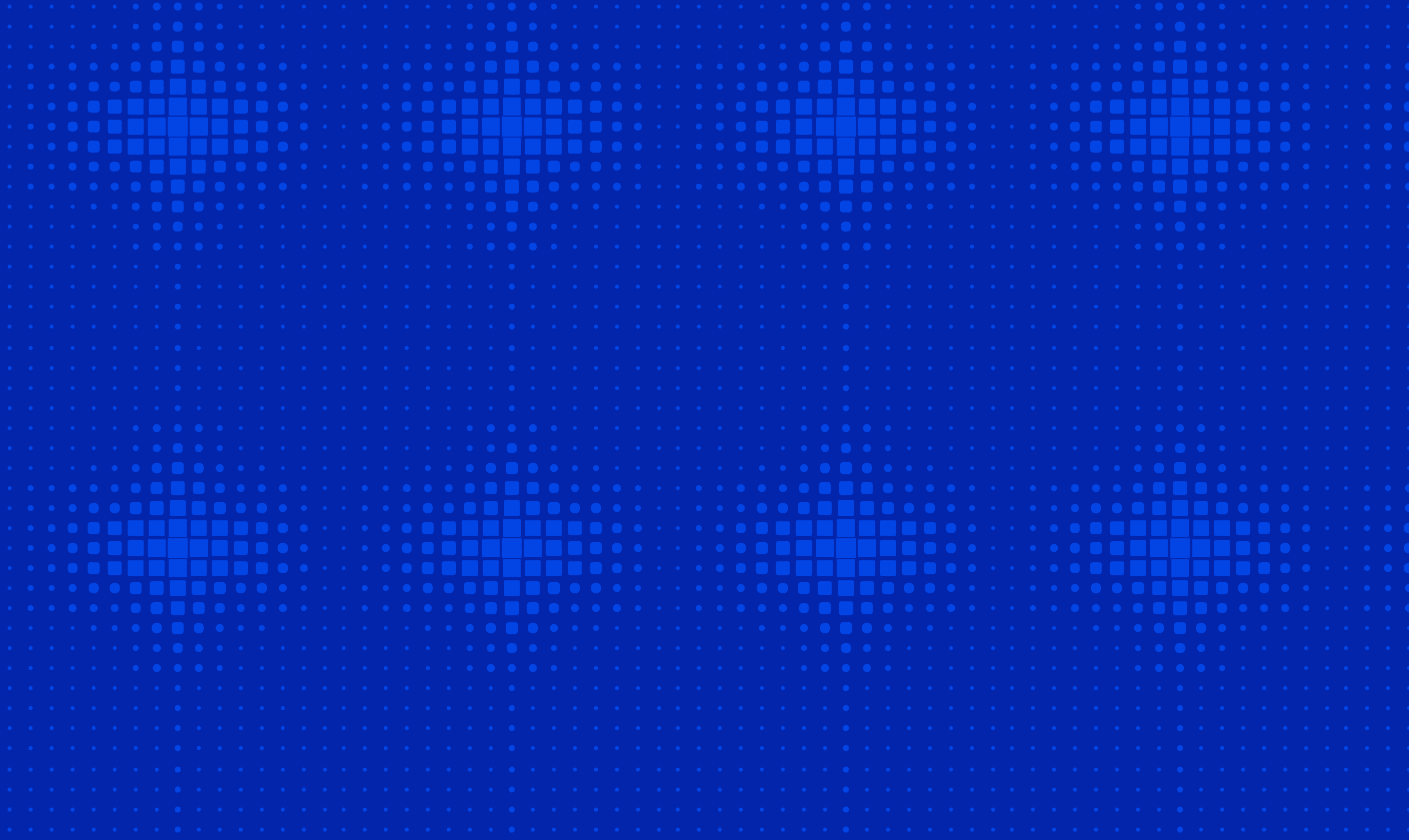
Workflow Orchestration
Successfully Deploying a Task Queue
December 2023

Prefect Product
Building an Application with Hashboard and Prefect
November 2023
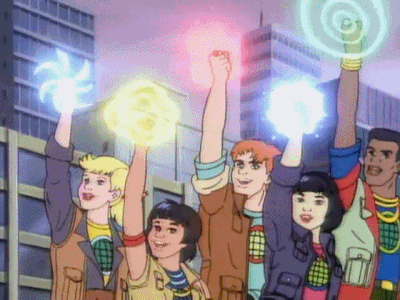
Workflow Orchestration
A platform approach to workflow orchestration
November 2023

Case Studies
Building a HIPAA compliant self-serve data platform
November 2023
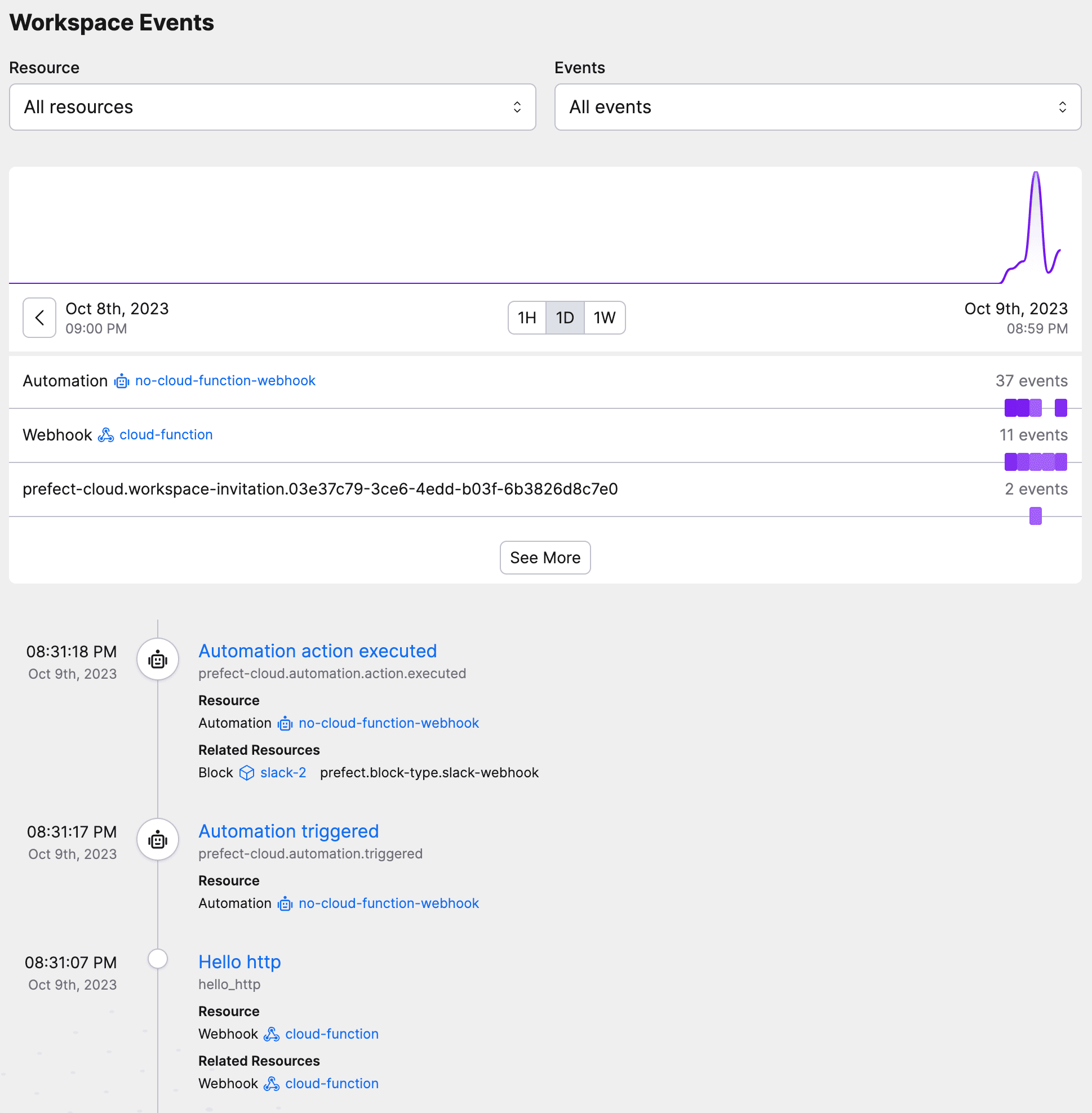
Prefect Product
Monitoring Serverless Functions: A Tutorial
November 2023
Prefect Product
Introducing access controls and team management
November 2023
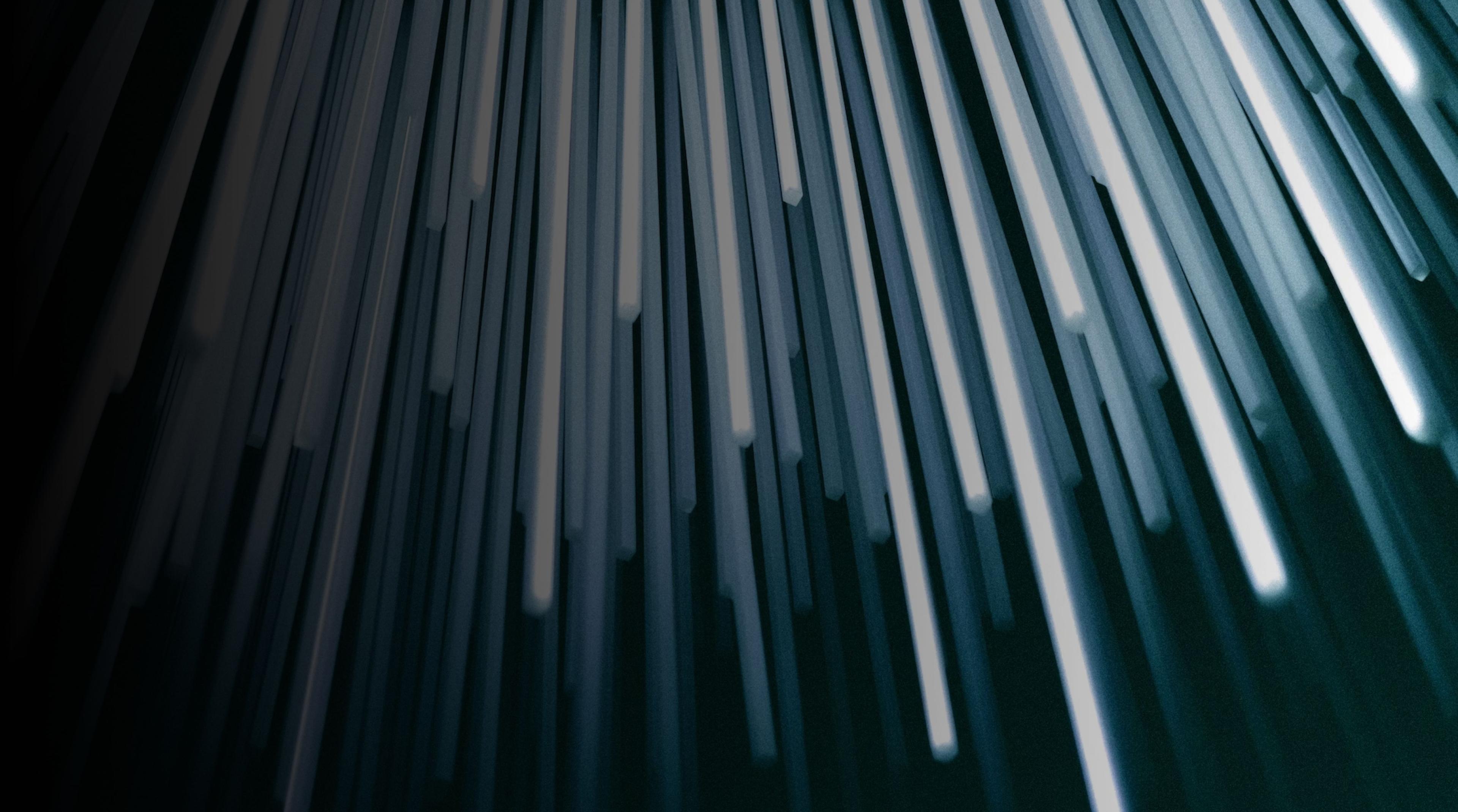
Engineering
Database Partitioning Best Practices
November 2023
Engineering
Building resilient data pipelines in minutes
October 2023
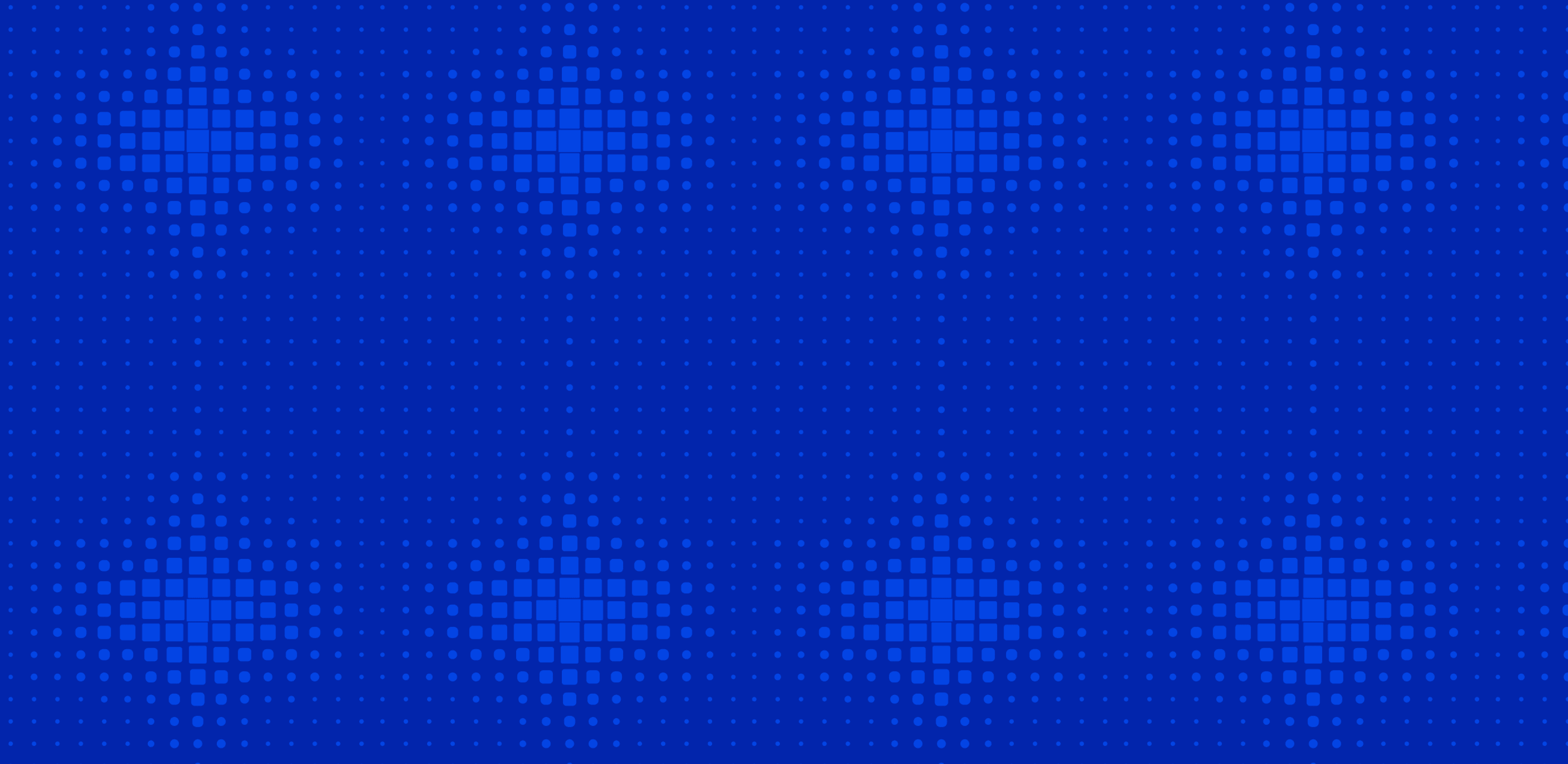
Workflow Orchestration
When to Run Python on Serverless Architecture
October 2023
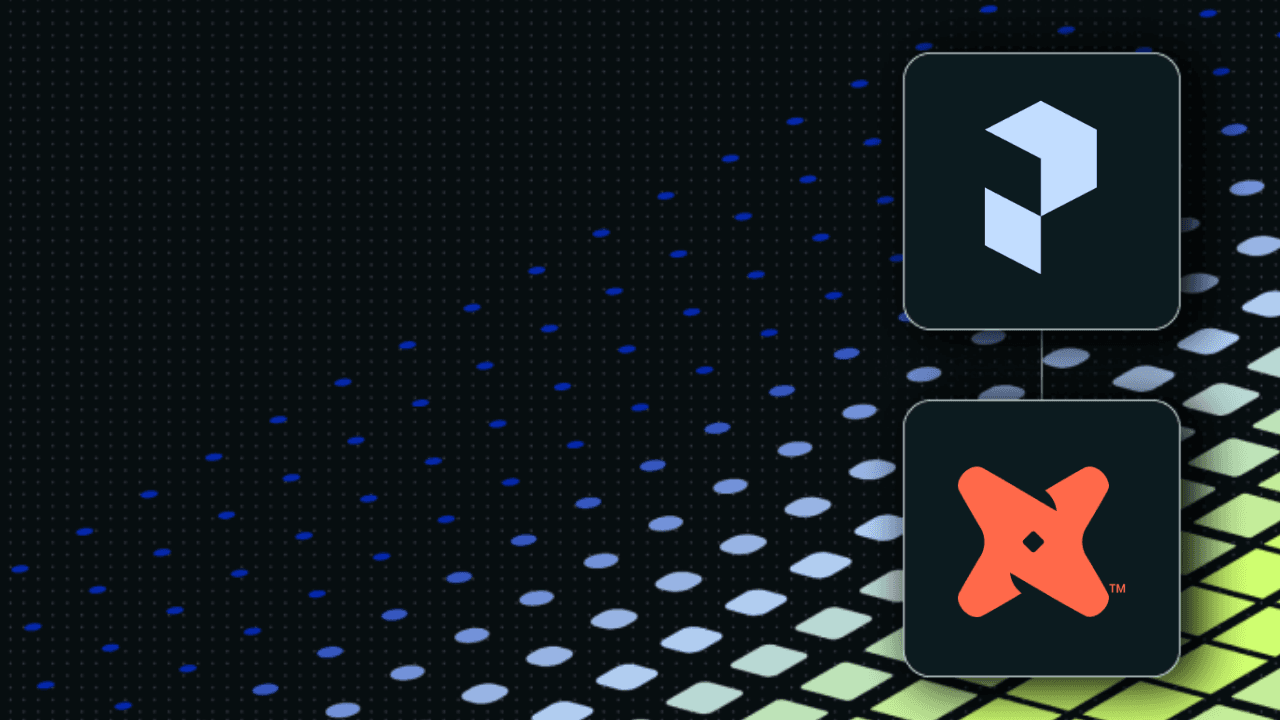
Prefect Product
dbt and Prefect: A quick tutorial
October 2023

Case Studies
Austin FC Scores Big with Data: A Strategic Play with Prefect
October 2023
Prefect Product
Introducing Error Summaries by Marvin AI
September 2023

Case Studies
Rec Room: From Workflow Chaos to Orchestrated Bliss
September 2023

Workflow Orchestration
The Implications of Scaling Airflow
September 2023

Workflow Orchestration
Data is Mail, Not Water
September 2023
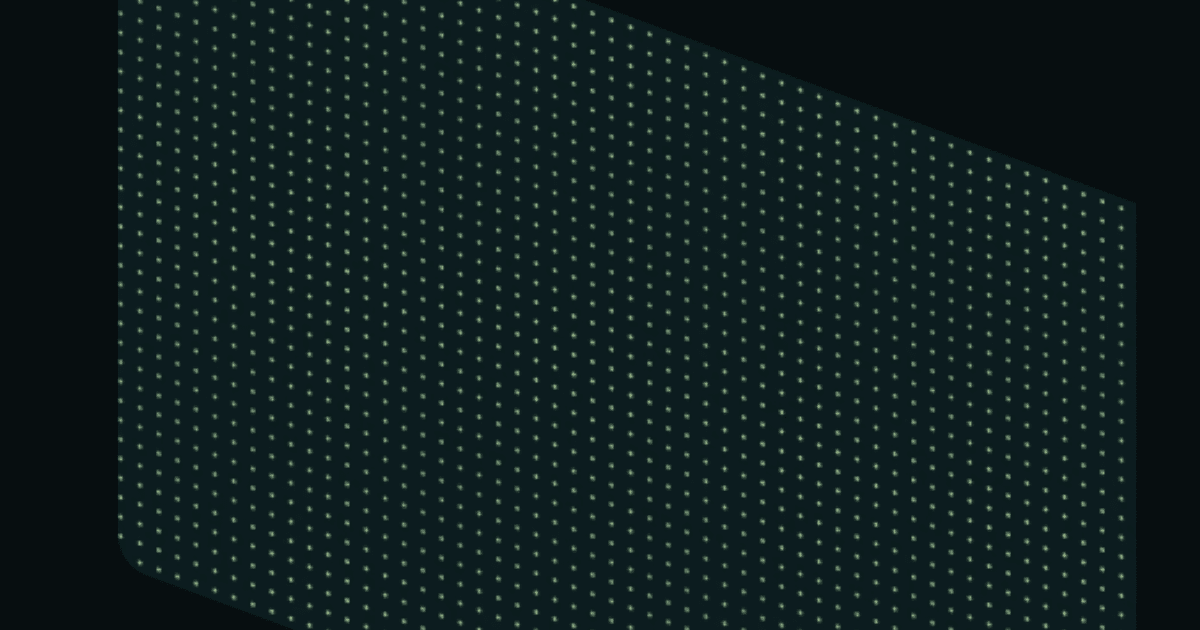
Prefect Product
Schedule your Python code quickly with .serve()
September 2023
Prefect Product
Automatically Respond to GitHub Issues with Prefect and Marvin
September 2023
Workflow Orchestration
What is a Data Pipeline?
September 2023
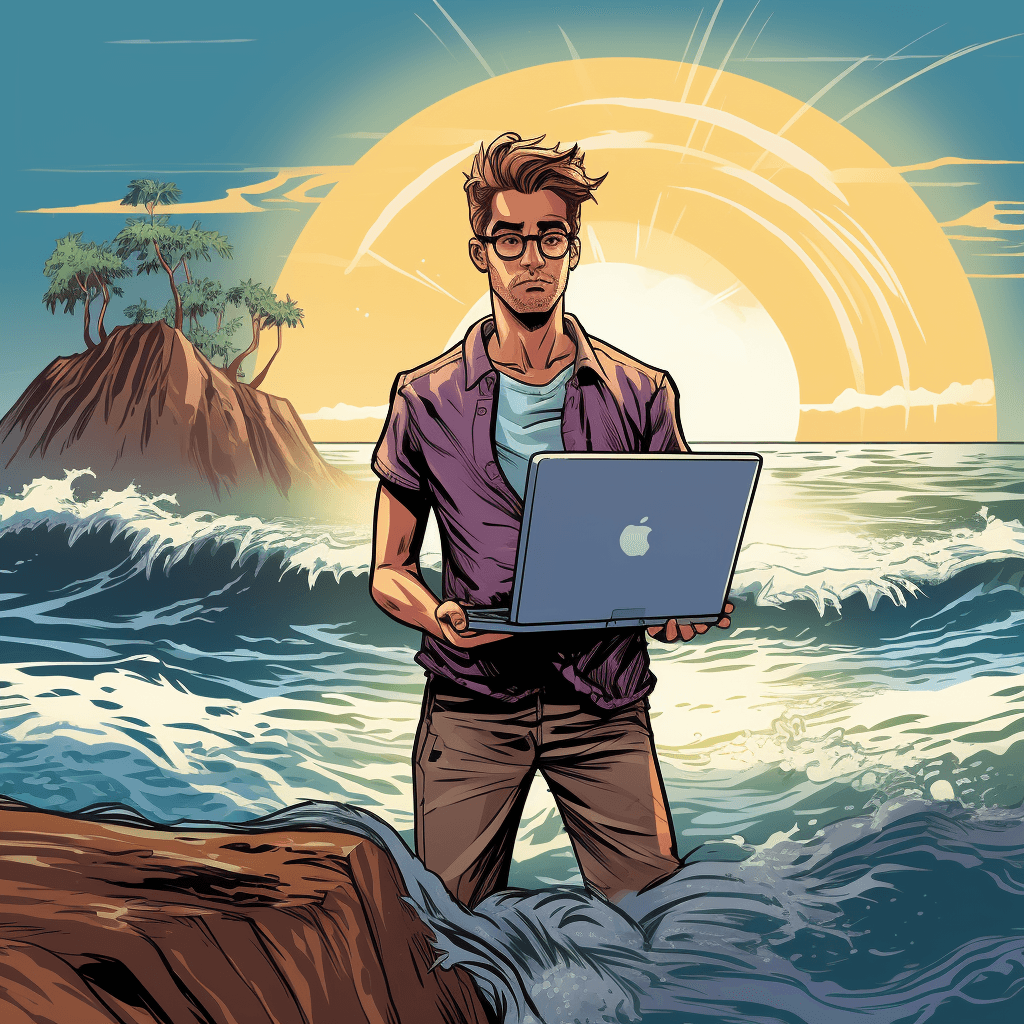
Workflow Orchestration
No Flow is an Island
August 2023
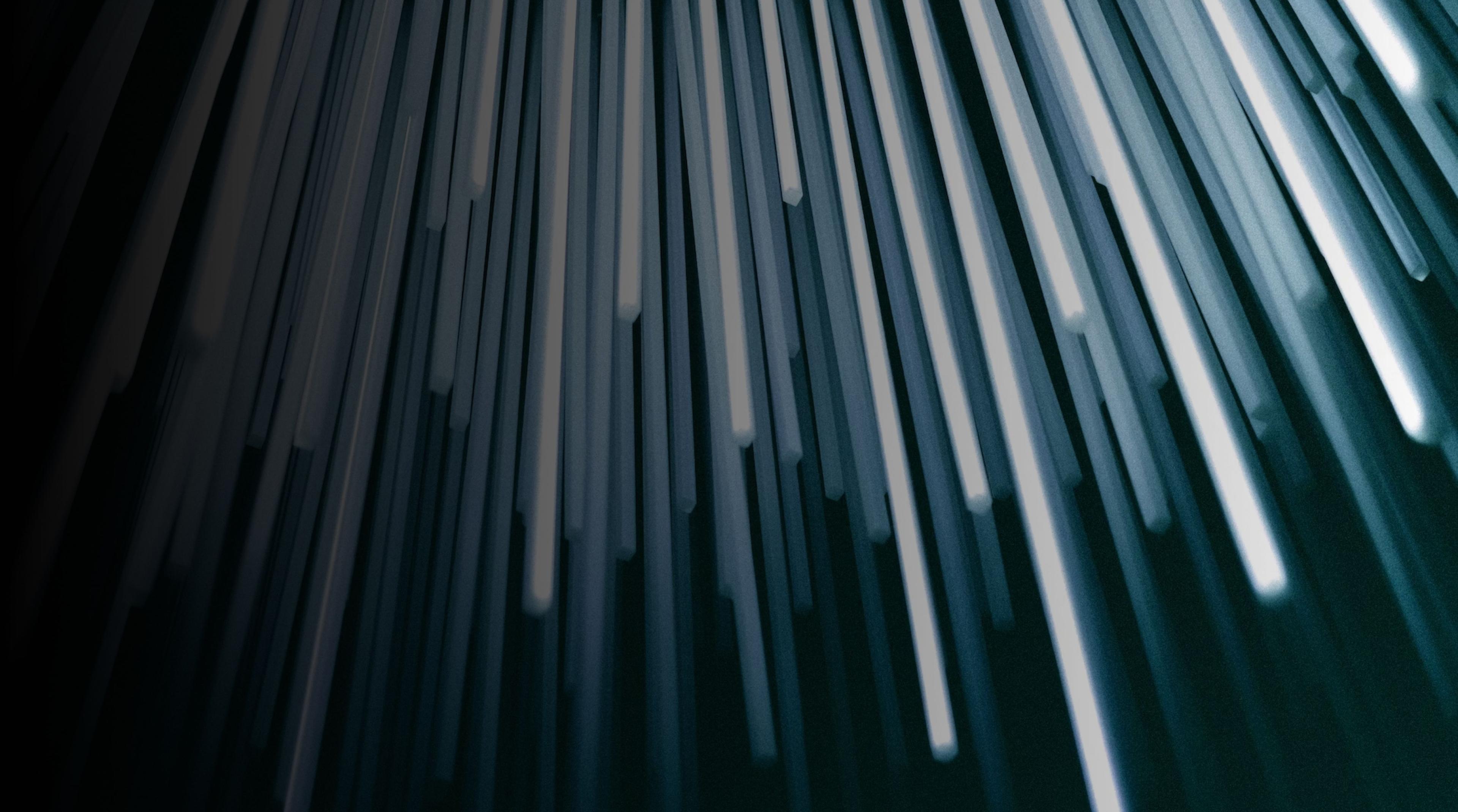
Prefect Product
Glue it all together with Prefect
August 2023
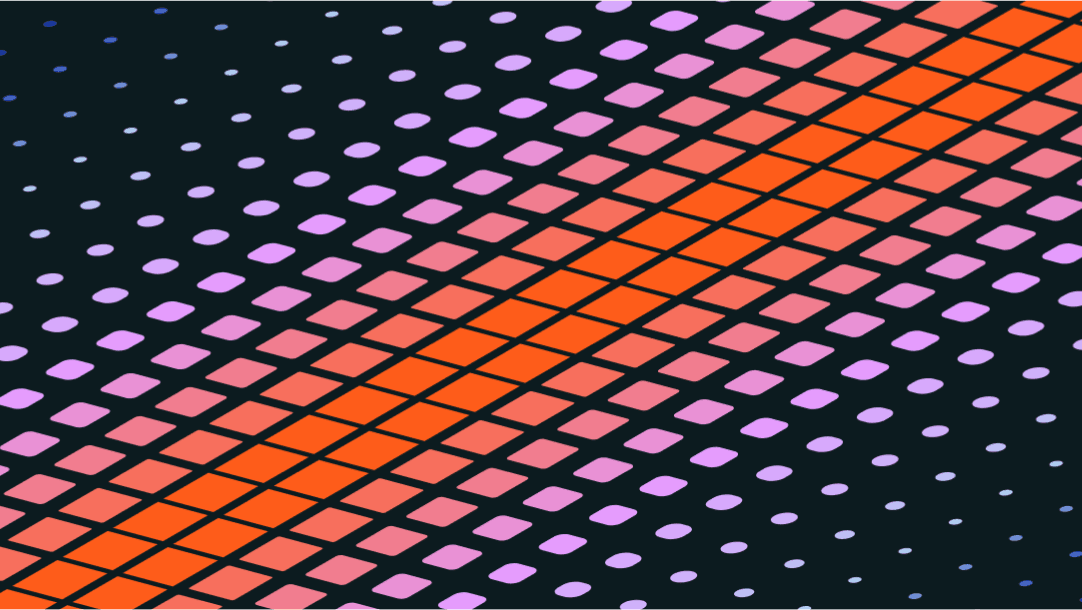
Workflow Orchestration
You Probably Don’t Need a DAG
August 2023
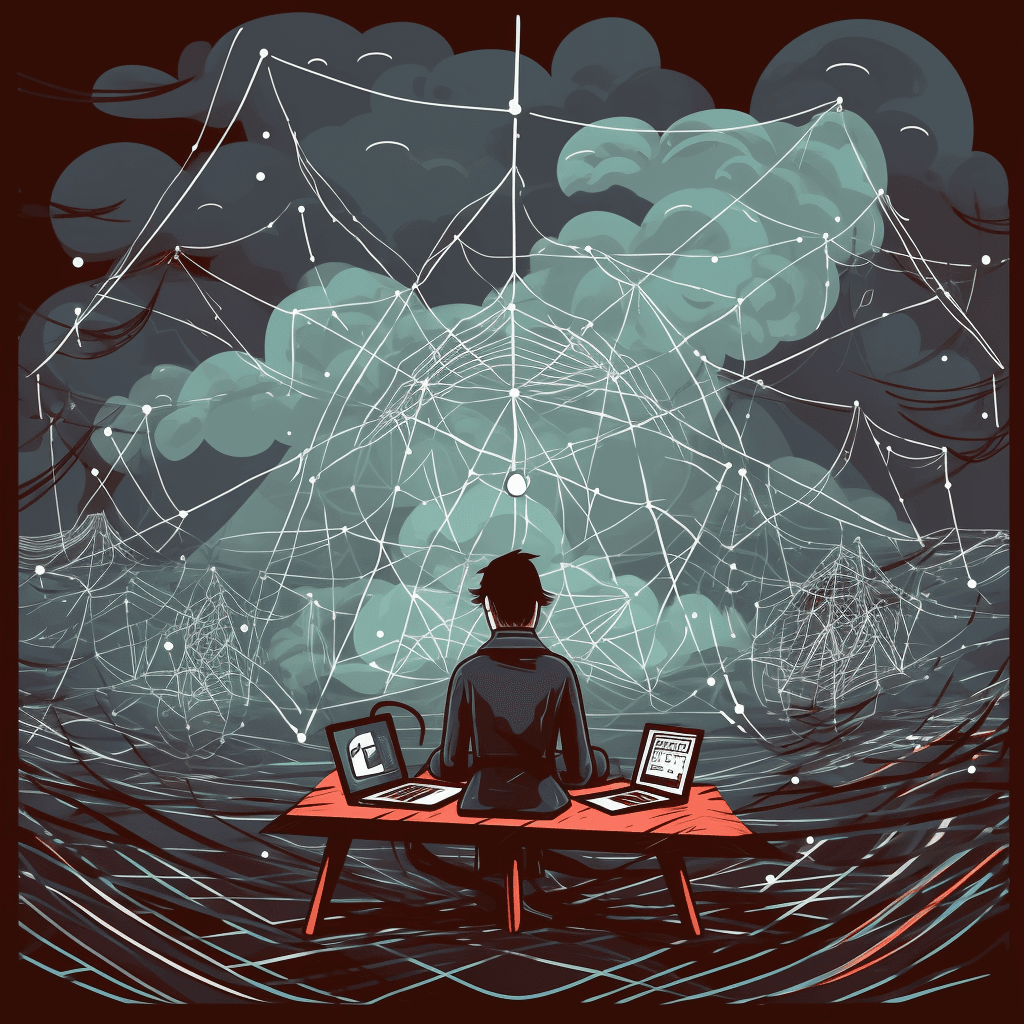
Workflow Orchestration
An Introduction to Workflow Orchestration
August 2023

Case Studies
Crumbl and Prefect: A Five Star Recipe
July 2023
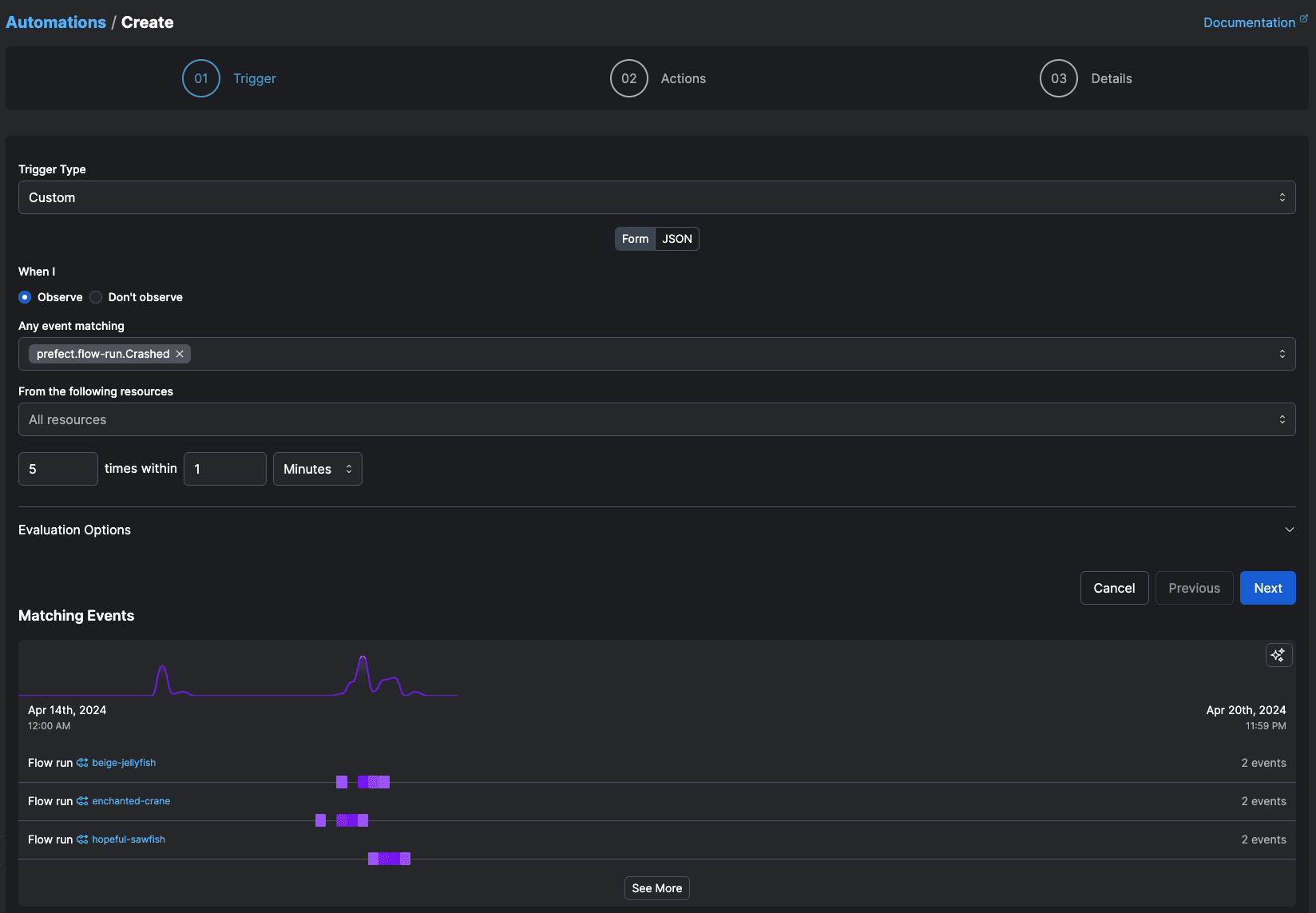
Prefect Product
Beyond Scheduling
July 2023
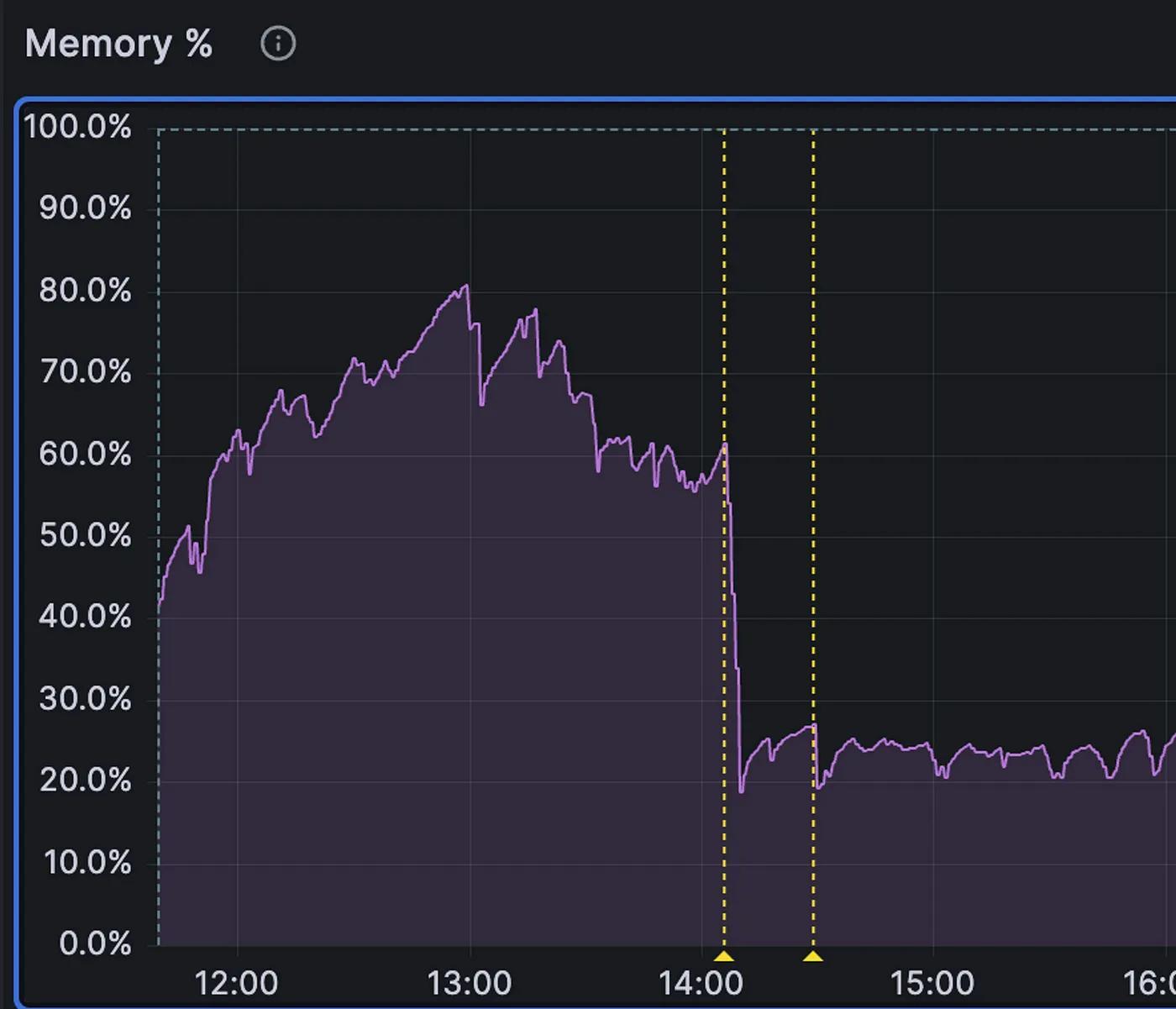
Engineering
More Memory, More Problems
May 2023
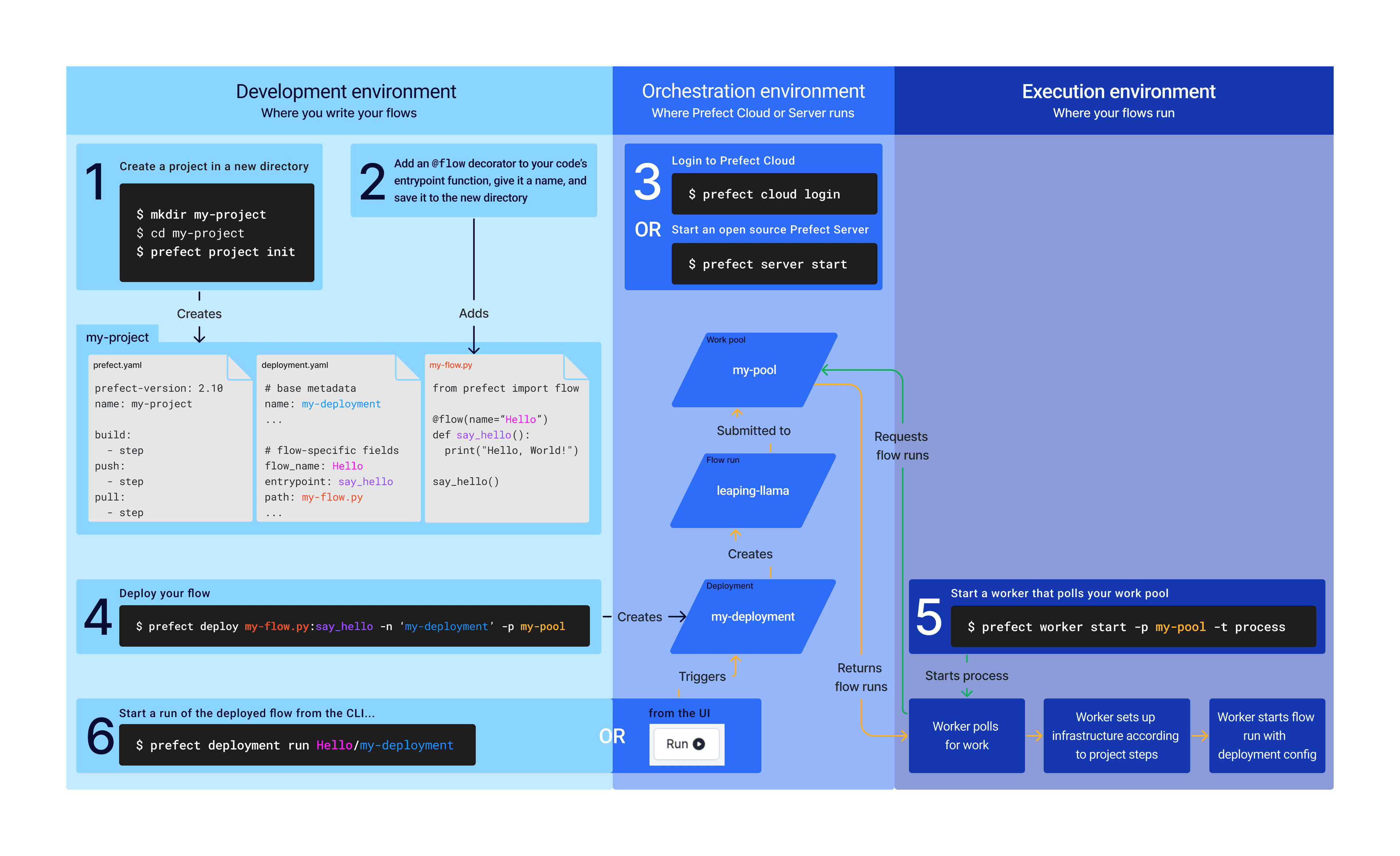
Prefect Product
Introducing Prefect Workers and Projects
April 2023
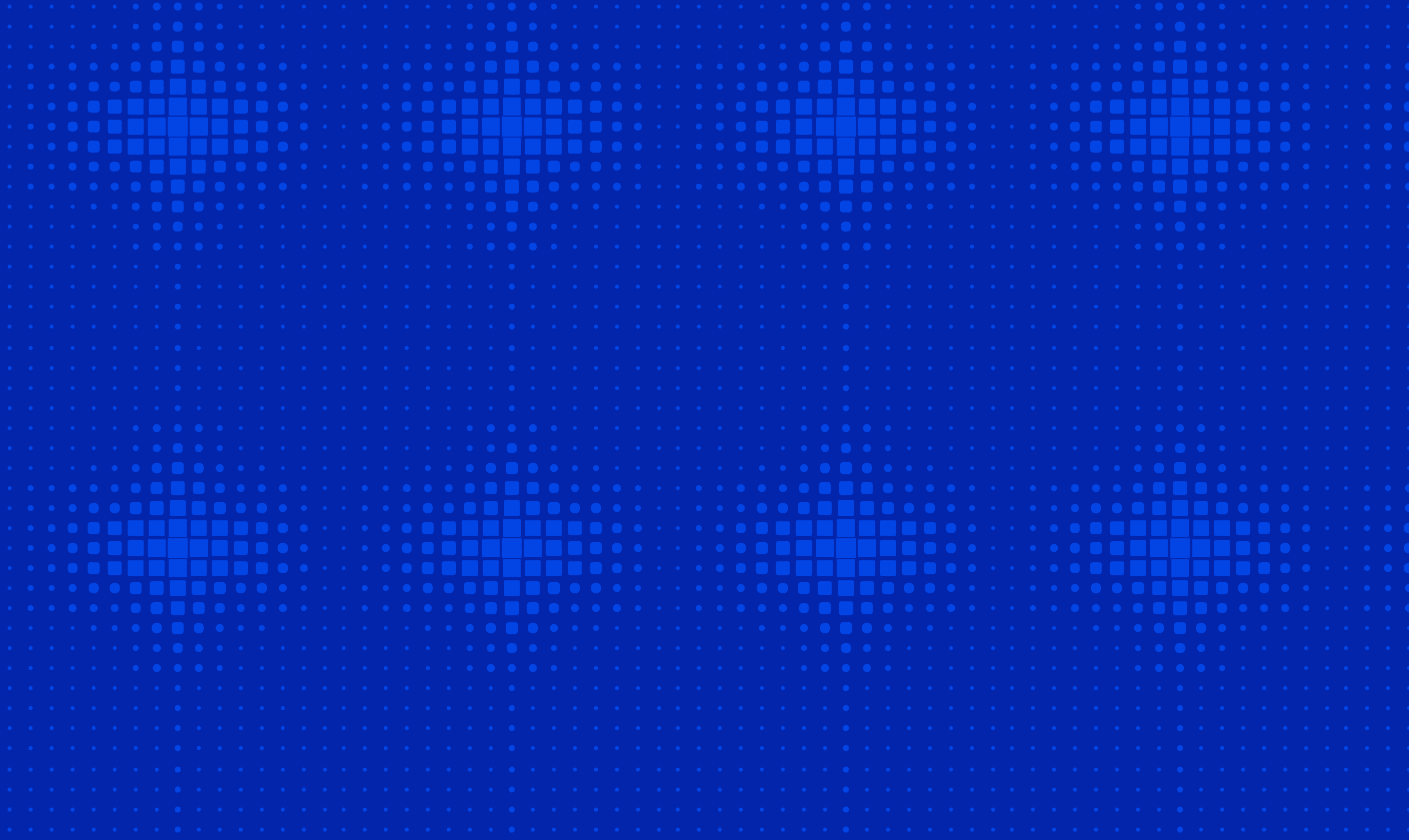
AI
Keeping Your Eyes On AI Tools
March 2023
Prefect Product
Freezing Legacy Prefect Cloud 1 Accounts
January 2023

Prefect Product
Workflow Design Patterns
January 2023
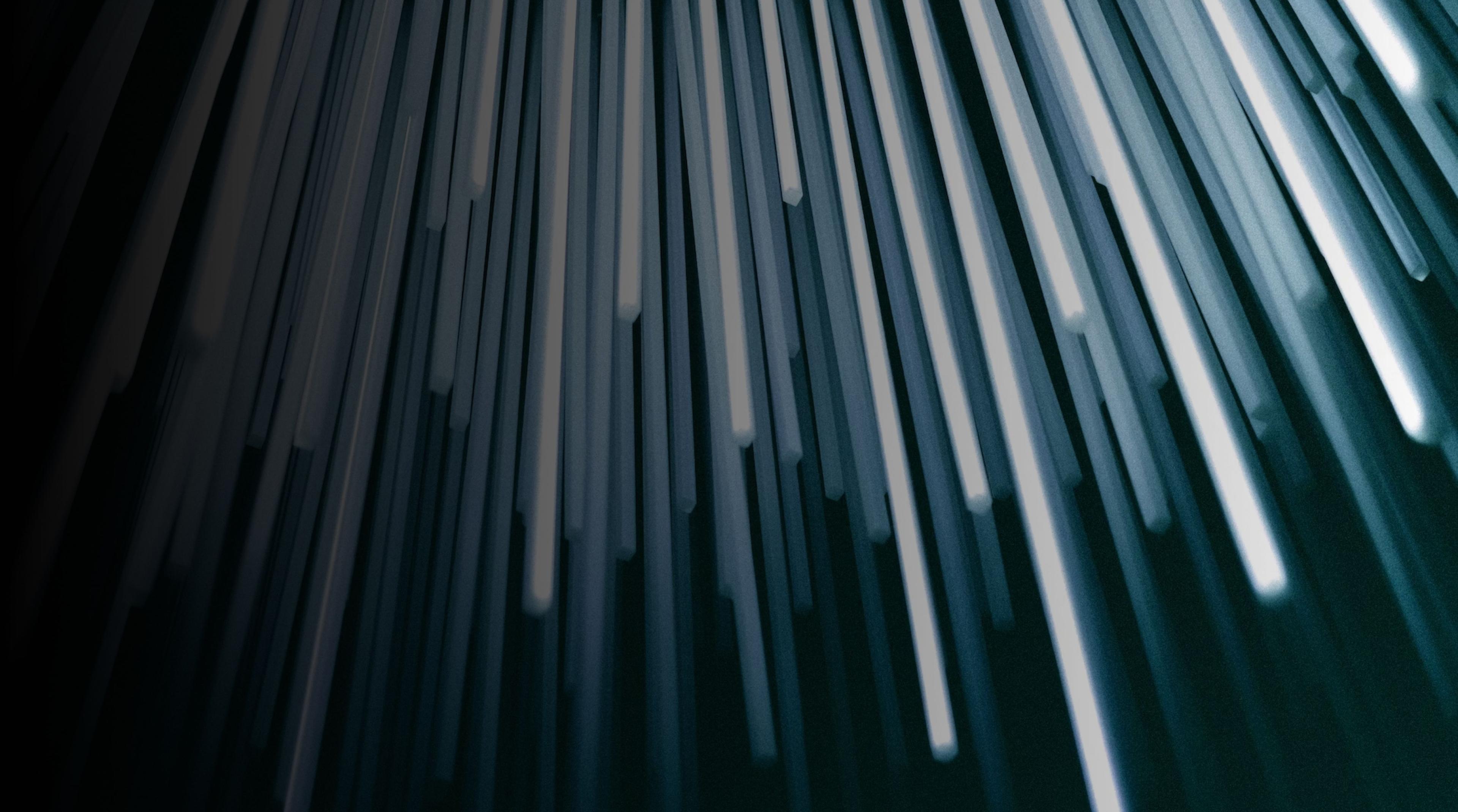
Prefect Product
Expect the Unexpected
January 2023

Case Studies
Anaconda and Prefect
December 2022
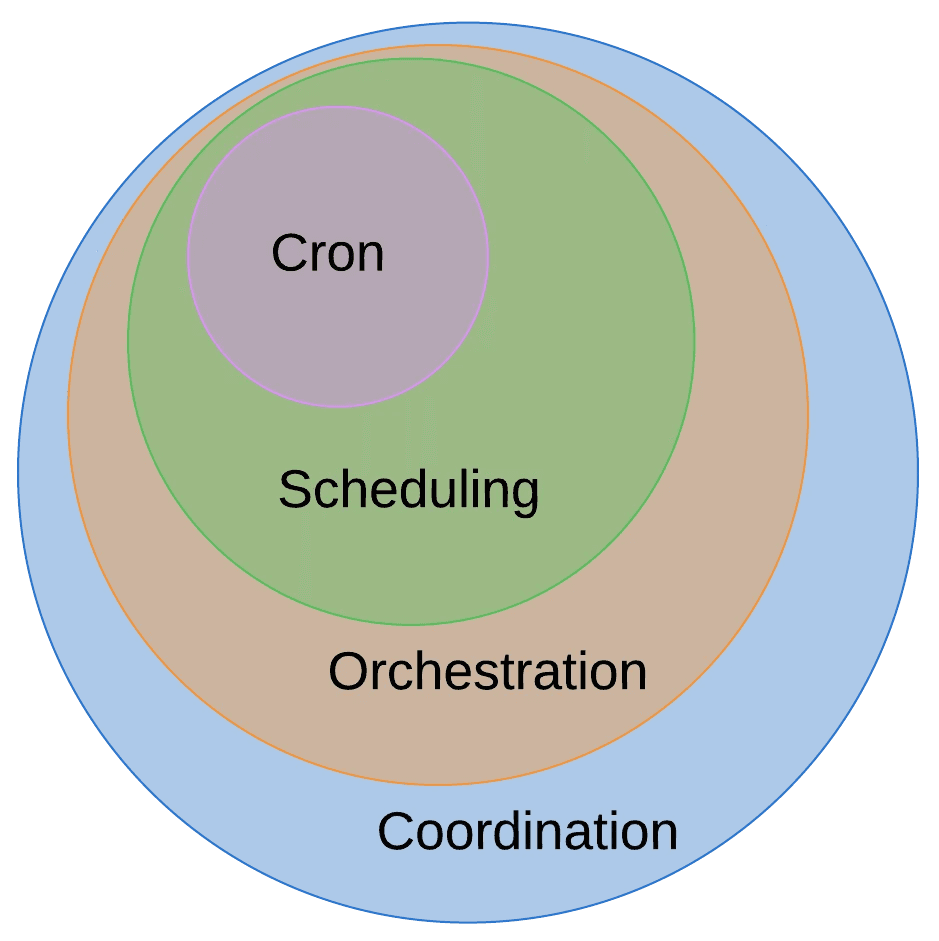
Workflow Orchestration
Why Not Cron?
October 2022
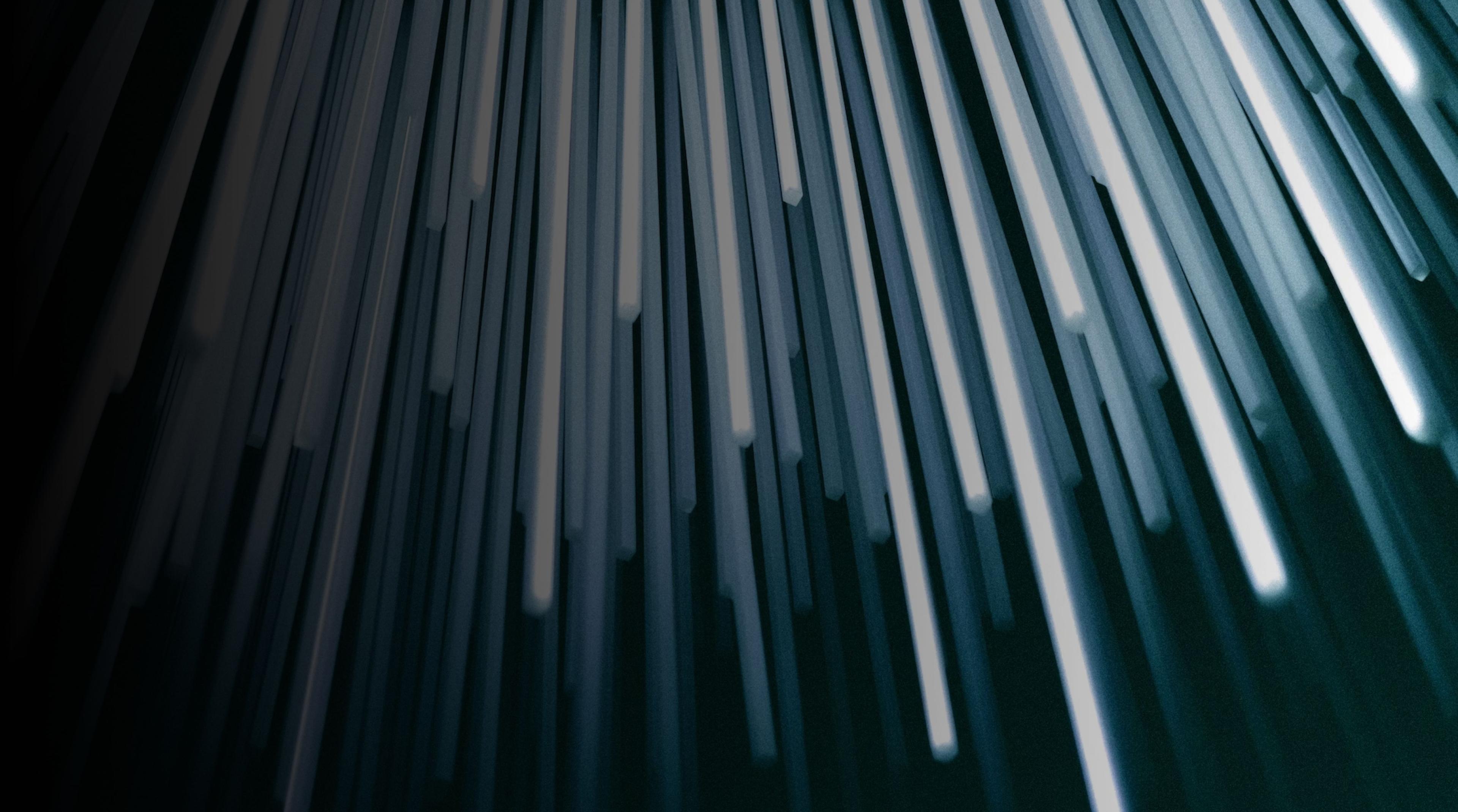
Workflow Orchestration
The Workflow Coordination Spectrum
September 2022
Prefect Product
If You Give a Data Engineer a Job
September 2022

Case Studies
Why Dyvenia Adopted Prefect
August 2022
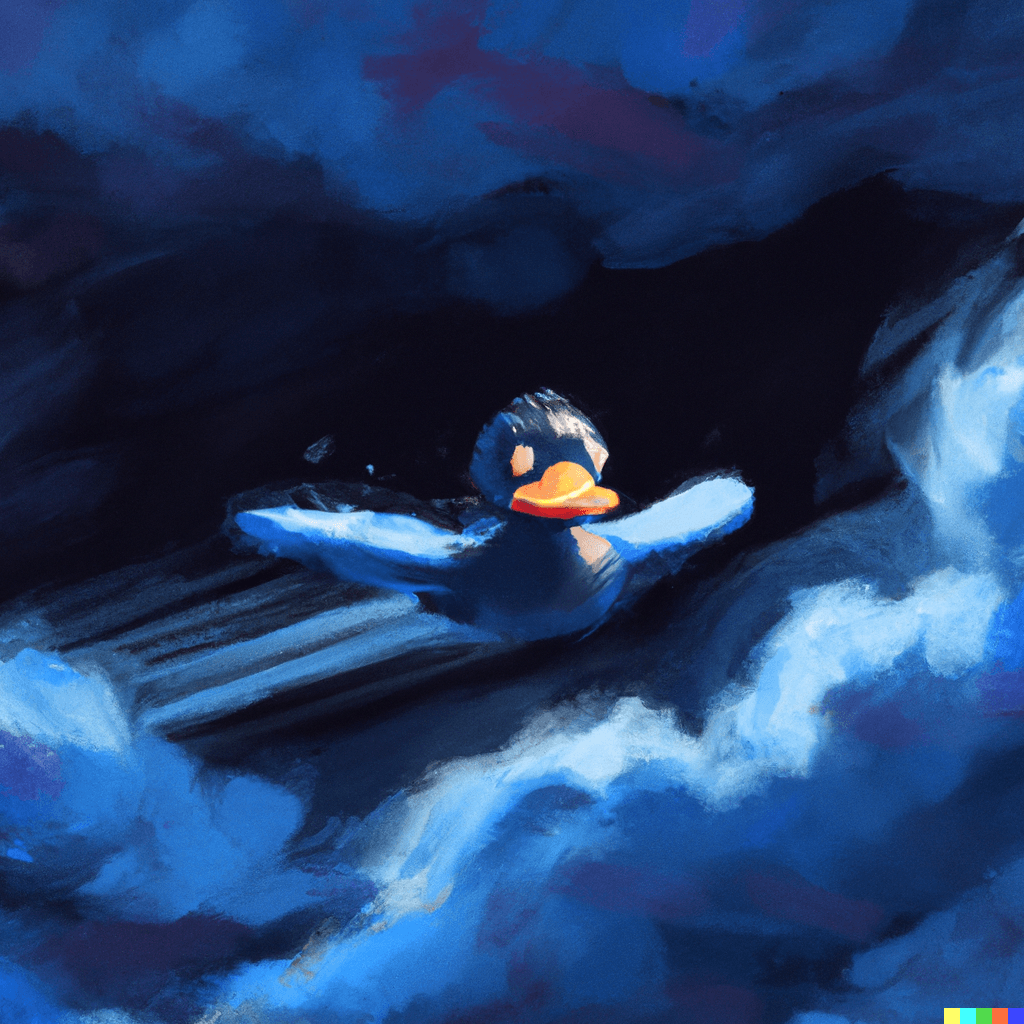
Prefect Product
(Re)Introducing Prefect
July 2022

Case Studies
RTR Struts the Data Runway with Prefect
May 2022
Workflow Orchestration
A Brief History of Workflow Orchestration
April 2022

Case Studies
Washington Nationals and Prefect
February 2022
Prefect Product
Our Second-Generation Workflow Engine
October 2021

Case Studies
Actium Health Produces Breakthrough Machine Learning Models with Prefect
September 2021

Press
Don't Panic: The Prefect Guide to Building a High Performance Team
April 2021

Case Studies
Clearcover Accelerates Data Insights With Prefect
November 2020

Workflow Orchestration
Why Not Airflow?
April 2019
Prefect Product
The Golden Spike
January 2019